A strategic approach to AI sustainability in South Africa
By Industry Contributor 17 December 2024 | Categories: feature articles.jpg)
By President Ntuli, Managing Director of HPE South Africa
With the AI race in full swing, many organisations now find themselves hoarding data in the hopes they will soon find a way to derive value from it.
Generative AI has the power to create personalised, data-driven content for millions in South Africa, creating a groundbreaking opportunity to tackle many local industry challenges. Although not a cure-all, AI shows immense promise across a number of vital sectors, including healthcare, education, financial services, and agriculture, potentially alleviating many of the issues these fields currently face.
Everyone knows AI is the solution – many of these industries have already proven as much – promising lower operating costs, increased innovation, and more.
Getting there the right way is the challenge. Like all technologies, AI must be efficiently designed and managed to avoid significant financial, environmental, and societal costs.
AI’s hunger for energy is immense… and growing
While AI represents a mere 2% of the global data centre footprint, the Uptime Institute predicts this will skyrocket to 10% of the sector’s global energy use by 2025.
AI requires a lot of energy, and one of the most energy-intensive portions of the AI lifecycle happens during model training. This training, typically performed on high-performance computing systems with thousands of accelerators, can take weeks or months to complete. Although specific details have not been disclosed, it is estimated that GPT-4, one of the most prominent generative AI models, consumed between 50 and 63GWh over a three-month training period. That’s more than 40 times what its predecessor, GPT-3, required.
But that’s just the initial investment in energy. The next energy-intensive portion of the AI lifecycle comes when the solution is asked to draw conclusions from the data, a phase commonly referred to as inference. For AI solutions like generative AI, inference energy use can dramatically exceed that consumed during the training stage due to the sheer number of queries that are processed.
We’re using AI all the time now. One major consumer AI solution launched in November 2022 and had 100 million active users two months later. And the cost of an AI query in terms of energy is about 15 times that of a standard search engine query.
Solving the sustainability dilemma
These energy demands aren’t sustainable in the long run – particularly given South Africa’s power supply challenges and the additional pressure AI could place on the grid.
So how do we design, deploy, and use AI to improve that?
First, technology leaders must determine if AI is the correct tool for the job. In other words: AI should not become a solution in search of a problem but rather part of a broader kit that is used only when it makes the most sense. As well, not every AI solution needs to be built from scratch. Organisations may not even need to develop or train an AI model from scratch, as many prebuilt and pre-trained models are now readily available.
The key to AI sustainability is developing a comprehensive AI business plan from the start. This includes addressing data needs, AI models, training and tuning requirements, as well as energy and cooling demands upfront.
To mitigate AI’s environmental impacts, leaders need a framework that optimises five key areas of efficiency. HPE technologists developed these five “levers” of sustainable technology, and they are especially relevant for AI solutions.
- Data efficiency: The effectiveness of AI solutions requires training and tuning with large amounts of data. Optimising the quality and volume of the data sets prior to beginning these equipment and energy-intensive processes can yield significant cost and energy savings. Data storage, retention, and disposition should also be considered when designing AI solutions to ensure that costs and value are balanced throughout the solution lifecycle.
- Software efficiency: Efficient AI model design can significantly reduce the hardware and energy requirements during training, tuning, and inference. The choice of programming language used for model development can also play a significant role in efficiency. Employing software engineering fundamentals of repeatability, reusability, and simplicity can further contribute significant efficiency benefits.
- Equipment efficiency: AI solutions often run best on high-performance hardware with specific acceleration, processing, and networking specifications. This hardware generally operates most efficiently at higher levels of utilisation that are common in AI solutions. Running AI solutions on equipment not optimised for these workloads often results in significantly higher space, energy, and cooling requirements with less efficient operation.
- Energy efficiency: Because AI solutions are so energy-intensive, performance per watt of energy is a key consideration when designing them. Increasingly, business requirements also mandate the use of renewable energy sources to power technology solutions to meet carbon reduction commitments. Locating AI solutions in facilities with 100% renewable energy available 24/7 remains a best practice.
- Resource efficiency: The energy intensity of the hardware required for AI solutions generates significant heat. In addition, the critical temperature of accelerators and other critical components is dropping, making effective cooling solutions vital. Close-coupled and direct liquid cooling enable high-performance AI solutions to function efficiently and effectively while minimising rack space required and facilitating heat reuse.
Incorporating each of these levers of sustainable IT into AI solution design, development, and implementation can lead to cost savings, less technological complexity, and a gentler environmental impact. In this way, efficiency is a key foundation for ensuring the effectiveness of AI solutions while balancing its overall impact on the planet.
Most Read Articles
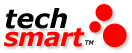

Have Your Say
What new tech or developments are you most anticipating this year?
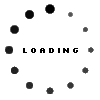