It's time to make AI boring
By Industry Contributor 14 January 2022 | Categories: feature articles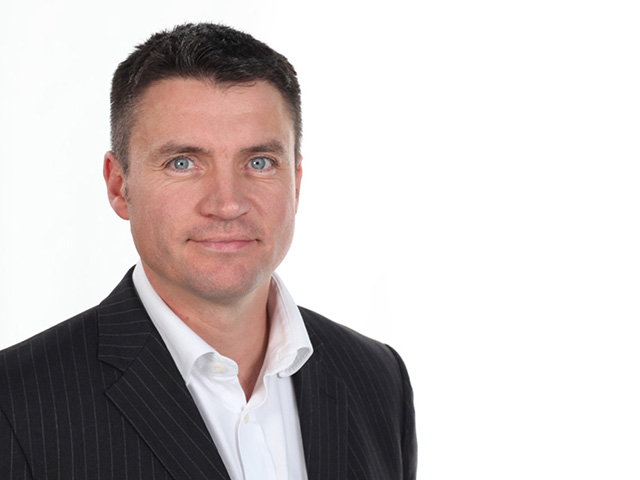
By Greg Newton, South Africa country manager, Blue Prism
The conversation about applying artificial intelligence (AI) in businesses has shifted dramatically. Not that long ago, organisations were being urged to “just get started”. To set up a dream team of data scientists, protect them from the rest of the company and let them get on with developing proofs of concept and failing or succeeding fast.
Those carefree days are over. The time has come to operationalise AI across the organisation at scale to start deriving clear business benefits, including improved customer service, and ROI from the time and money already spent. Beyond that, the pandemic has undoubtedly accelerated digitalisation – compressing years into months – and data-first, AI-enabled organisations are the ones that are going to succeed in the future.
In a way, AI now needs to become boring. It needs to become part of the fabric of any organisation and not even be noteworthy anymore. It should be a foregone conclusion. We're a long way off from this though. And much of the conversation around operationalising AI has looked at AI and data strategies, partnerships, and ethical frameworks around scaling AI. All of these are of great importance, but they're missing the most important ingredient to ensuring technology uptake: humans.
And the conversations that are happening around humans and AI typically focus on reskilling and educating people about the synergy between humans and AI – that AI is an opportunity, not a threat, to their jobs. (I've written about the latter myself.) But we're still missing a critical aspect of the role humans need to play in driving AI across the organisation, and that is bridging the gap between data and business. While it made sense to separate out your AI innovation team from the rest of the company in the early days, we now need to fold data back into the business at an existential level.
This takes work and effort from both sides, but for AI to scale and operationalise across the organisation effectively and quickly, data and business need to become best friends so you can avoid some of the common reasons AI fails to scale.
Three reasons AI fails to scale and what you can do about it
- Data science/AI and business are disconnected. Companies need to stop thinking about AI and the business function as being distinct functions, for instance treating customer experience and AI as distinct entities. AI should be a fully paid-up, card-carrying member of the CX team. A disconnect here is a common reason for why AI does not successfully get pushed through an organisation. Data scientists need to be embedded into the functional teams, they need to learn how to speak the business language and understand the business strategy. Importantly, they need to be incentivised in the same way as the business they are serving, for example on revenue as well as project delivery, if that is how the rest of the business unit is incentivised. Only with everyone around the same table can AI scale.
- Building out the AI model is only the first step. When AI remains disconnected from the rest of the organisation, it is easy for data scientists to fail to realise that their code is only a small fraction of the overall system, and is very often the easiest part of the work. Their model has to be used in a real-world context by businesspeople, and building this is where 90% of the time, money and work will be spent. Ultimately, the business user also needs capabilities such as reporting, auditing, a user-friendly front-end and security for the AI model to drive their business results. The data scientists that are most successful are the ones who fully engage with the business side of their organisation. They keep asking questions and thinking through how their work is consumed by the business user or customer to genuinely understand the business they are serving.
- Your legacy systems are holding you back. Say you've managed to shepherd an AI-driven CX project through the organisation. Data and business have teamed up to evangelise the real-world business benefits of the project, demonstrated how these are aligned with the business objectives and not bamboozled business folk by throwing an algorithm up on a screen. The CX team is happy they'll be able to use the output in a way that works for them, and everyone is happy that adopting the functionality will improve customer experience and drive incremental revenue. But there's one more bridge to cross. To get the data into the AI model, and then the outputs onto the website, siloed legacy systems need to be completely overhauled, costing time and money you don’t have. In this case it’s robotic process automation (RPA)-powered digital workers that are the solution: use RPA to “manually” migrate the data, quickly and accurately, between the disparate systems and get your AI project off the ground anyway.
The world has shifted from “just get started” to the urgency to embed AI into your organisation’s core. I was only being slightly tongue-in-cheek when I said let’s make AI boring. We’ve only begun to scratch the surface of the capabilities AI offers, but a precondition for these emerging is to have AI threaded through our organisations as a mainstream technological capability.
Most Read Articles
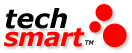

Have Your Say
What new tech or developments are you most anticipating this year?
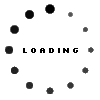